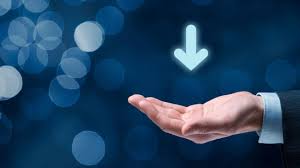
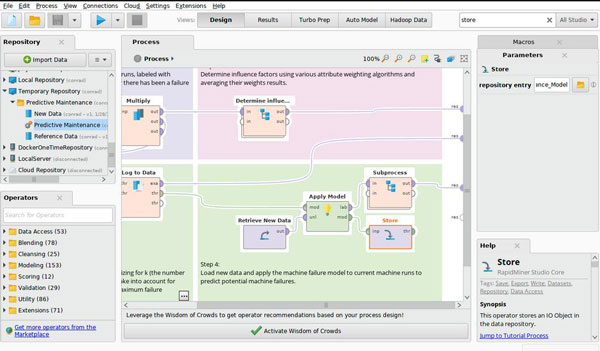
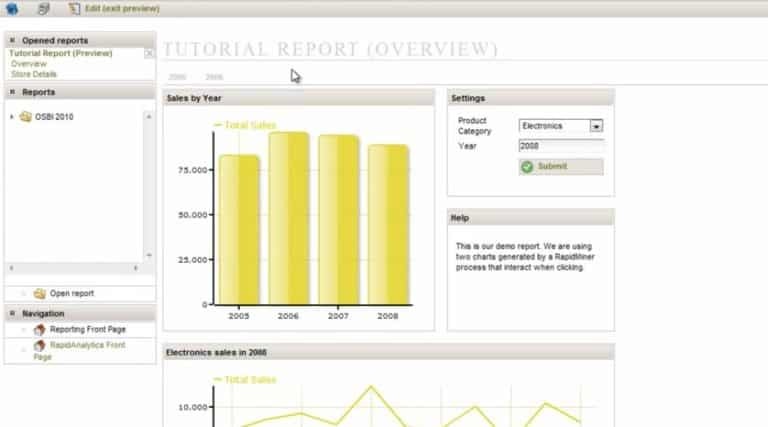
This unique approach is the only guarantee that no overfitting is introduced and no overestimation of prediction performances can occur RapidMiner Studio follows a stringent modular approach which prevents information used in pre-processing steps leaking from model training into the application of the model.Dimensionality reduction with Self- Organizing Maps (SOM).Choose the number of components to be retained.Calculates Eigenvalues and Eigenvectors from correlation and covariance matrices.Plots for principal components coefficients, Eigenvalues, and cumulative variance of Eigenvalues.– Removal of attributes unrelated to target based on arbitrary weighting schemes like information gain, Gini index, and others – Removal of attributes unrelated to target based on a chi-square or correlation-based selection criterion – Selection of attributes by user specification – 30+ weighting schemes measuring the influence of attributes & forming base or weight-based selections (filter approach) Miscellaneous functions: if then-else, square root, signum, random, modulus, sum, binomial, missing binomial, missing.
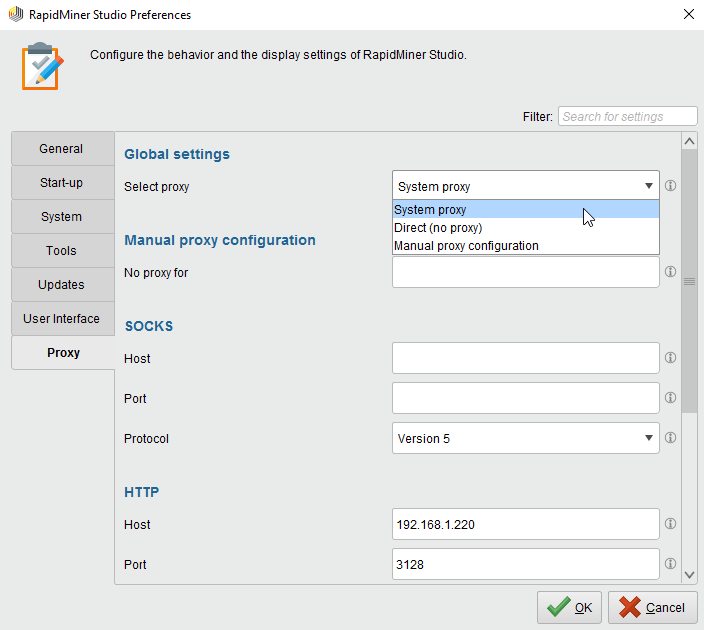

#Res in rapidminer studio series#
Access to time series data, audio files, images, and many more.Connect to Zapier and trigger Zapier tasks.Repository-based data management on local systems or central servers via RapidMiner Server.Access to full-text index & search platform SOLR.Support for all JDBC database connections including Oracle, IBM DB2, Microsoft SQL Server, MySQL, Postgres, Teradata, Ingres, VectorWise, and more.Access to text documents and web pages, PDF, HTML, and XML.Access to Cloud storage like Dropbox and Amazon S3.Access to NoSQL databases MongoDB and Cassandra.Wizards for Microsoft Excel & Access, CSV, and database connections.Access to more than 40 file types including SAS, ARFF, Stata, and via URL.Also transform unstructured data into structured. Access, load and analyze any type of data – both traditional structured data and unstructured data like text, images, and media.
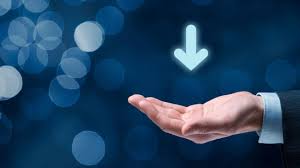